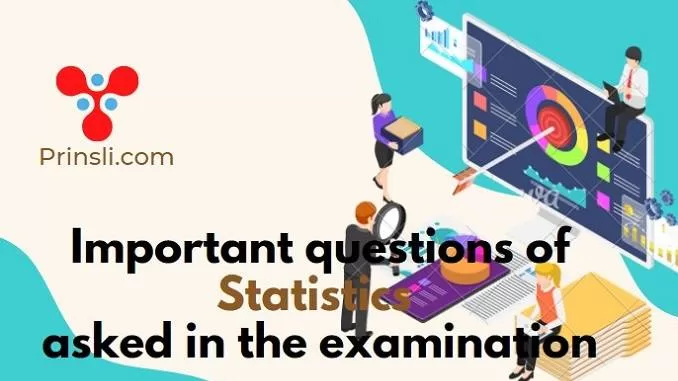
List of 100 important questions of Statistics asked in the entrance examination for Postgraduate admission –
I can’t give you exact questions from past exams. But I can provide you with many example questions covering various topics in Statistics. These important questions of statistics are commonly asked in entrance exams for postgraduate programs. Here’s a selection of 100 important questions across different areas of Statistics:
Descriptive Statistics:
- Define statistics and explain its role in data analysis.
- Discuss the difference between descriptive and inferential statistics.
- Explain the types of data and measurement scales used in statistics.
- Describe the steps involved in the statistical investigation process.
- What is the difference between a population and a sample?
- Define measures of central tendency and provide examples.
- Explain the concept of variability in statistics.
- Describe the properties of the normal distribution.
- What is the interquartile range, and how is it calculated?
Correlation Analysis:
- Define correlation and discuss its interpretation.
- Explain the concept of correlation and discuss its types.
- Calculate Pearson’s correlation coefficient for a given dataset.
- Describe the Pearson correlation coefficient and its interpretation.
- Discuss the Spearman rank correlation coefficient and its properties.
Regression Analysis:
- Explain the concept of regression analysis and its applications.
- Define simple linear regression and its components.
- Explain the concept of linear regression and its assumptions.
- Discuss the assumptions underlying linear regression.
- Discuss the difference between simple linear regression and multiple regression.
- Describe the least squares method for estimating regression coefficients.
- Explain the concept of coefficient of determination and its interpretation.
- Discuss the assumptions of regression analysis and how to check them.
- Interpret the coefficients of a linear regression model.
- Interpret the slope and intercept coefficients in a regression model.
- Describe the process of model selection in regression analysis.
- Explain how to assess the goodness of fit of a regression model.
Correlation and Regression Analysis:
- Discuss the difference between correlation and regression analysis.
Logistic Regression:
- Define logistic regression and its applications.
- Discuss the assumptions of logistic regression and how to check them.
- Explain the logistic function and its interpretation.
- Interpret the odds ratio in logistic regression.
- Discuss the assumptions of logistic regression.
- Compare logistic regression to other classification methods.
- Describe the likelihood ratio test and its use in logistic regression.
- Explain the concept of odds ratio and its interpretation in logistic regression.
- Discuss the use of ROC curves in evaluating the performance of logistic regression models.
Time Series Analysis:
- Define a time series and discuss its components.
- Define time series analysis and discuss its components.
- Describe different methods for forecasting time series data.
- Explain the concepts of trend, seasonality, and cyclical variations in time series.
- Explain the concept of stationarity in time series analysis.
- Explain the importance of stationarity in time series analysis.
- Describe different methods for decomposing a time series.
- Describe the methods for smoothing time series data, such as moving averages and exponential smoothing.
- Discuss autoregressive integrated moving average (ARIMA) models.
- Discuss the autoregressive integrated moving average (ARIMA) model and its applications.
- Discuss the Box-Jenkins methodology for fitting ARIMA models.
- Discuss the autocorrelation function (ACF) and its interpretation.
- What is seasonality, and how is it addressed in time series analysis?
Probability Theory:
- Define probability and discuss its interpretation.
- Explain the difference between independent and mutually exclusive events.
- Calculate the probability of compound events using the addition and multiplication rules.
- Discuss the concept of conditional probability.
- What is Bayes’ theorem, and how is it applied in probability theory?
- Explain the concept of probability and its role in statistical inference.
Random Variables and Probability Distributions:
- Define probability distribution and discuss its properties.
- Define a random variable and distinguish between discrete and continuous random variables.
- Discuss the difference between discrete and continuous random variables.
- Explain the concept of expected value and variance for a random variable.
- Explain the characteristics of the binomial distribution.
- Discuss the properties of the binomial distribution and its applications.
- Discuss the properties of the Poisson distribution and its applications.
- Describe the Poisson distribution and its properties.
- Define the exponential distribution and describe its probability density function.
- What is the standard normal distribution, and how is it used in statistical inference?
- Explain the concept of the normal distribution and its importance in statistics.
Sampling and Estimation:
- Explain the concept of sampling and its importance in statistics.
- Define sampling bias and discuss its implications for statistical inference.
- Describe different sampling techniques, such as simple random sampling and stratified sampling.
- Discuss the concept of point estimation and provide examples.
- Explain the difference between a parameter and a statistic.
- Define population, sample, and parameter, and discuss their relationship.
Sampling Techniques:
- Discuss the importance of sampling techniques in statistical analysis.
- Define simple random sampling and discuss its advantages.
- Explain stratified sampling and its use in population subgroups.
- Discuss cluster sampling and its applications.
- What is systematic sampling, and how is it implemented?
- Compare and contrast probability and nonprobability sampling methods.
- Discuss the Central Limit Theorem and its implications for sampling distributions.
- Explain the concept of sampling distribution and its importance in inferential statistics.
- Discuss the difference between point estimation and interval estimation.
Hypothesis Testing:
- Define hypothesis testing and explain its steps.
- Define null and alternative hypotheses.
- Discuss the steps involved in hypothesis testing.
- Explain Type I and Type II errors in hypothesis testing.
- Calculate a test statistic for a given hypothesis test.
- What is the significance level, and how is it related to the p-value?
- Explain the concept of p-value and its interpretation in hypothesis testing.
- Describe the difference between one-tailed and two-tailed tests.
- Define statistical power and discuss its relationship with Type II error.
- Explain the concept of effect size and its importance in hypothesis testing.
- Discuss the factors that affect the power of a statistical test.
- Define the t-distribution and discuss its properties.
- Explain the concept of degrees of freedom and its importance in hypothesis testing.
Parametric Tests:
- Discuss the z-test and t-test for population means and their assumptions.
- Discuss the t-test and its applications in comparing means.
- Describe the assumptions underlying the t-test.
- Explain the one-sample and independent samples t-tests.
- What is the paired samples t-test, and when is it used?
- Define analysis of variance (ANOVA) and discuss its use in comparing multiple groups.
Nonparametric Tests:
- Define nonparametric statistics and discuss its advantages and limitations.
- Explain the Mann-Whitney U test and its applications.
- Describe the Kruskal-Wallis test and its use in comparing multiple groups.
- Discuss the Wilcoxon signed-rank test and its applications.
- What is the sign test, and how is it used in nonparametric statistics?
- Explain the chi-square test for independence and its assumptions.
- Explain the concept of goodness-of-fit tests and its applications.
- Discuss the chi-square goodness-of-fit test and its assumptions.
- Define the Kolmogorov-Smirnov test and its use in testing for normality.
- Explain the concept of outlier detection and its methods.
- Discuss the use of boxplots and scatterplots in identifying outliers.
- Define robust statistics and discuss its applications in handling outliers.
- Describe the concept of data transformation and its use in handling non-normal data.
Multivariate Analysis:
- Define multivariate analysis and discuss its applications.
- Explain principal component analysis (PCA) and its use in dimensionality reduction.
- Describe factor analysis and its applications in identifying latent variables.
- Discuss discriminant analysis and its use in classification problems.
- What is cluster analysis, and how is it used in data analysis?
- Discuss applications of cluster analysis in grouping similar observations.
- Define survival analysis and discuss its applications.
- Describe Kaplan-Meier survival curves and their interpretation.
- Explain the concept of censoring in survival analysis.
- Discuss the log-rank test and its use in comparing survival curves.
Probability Distributions:
- Define probability distribution and discuss its types.
- Define probability distributions for continuous random variables, such as the uniform, exponential, and normal distributions.
- Explain the properties of the normal distribution.
- Describe the standard normal distribution and its properties.
- Discuss the characteristics of the binomial distribution.
- Describe the Poisson distribution and its applications.
- What is the exponential distribution, and when is it used?
- Discuss the properties of the exponential distribution and its applications.
Confidence Intervals:
- Define a confidence interval and its interpretation.
- Discuss the factors that affect the width of a confidence interval.
- Explain the concept of margin of error in confidence intervals.
- Calculate a confidence interval for a population mean.
- Explain how sample size affects the width of a confidence interval.
- What is the margin of error, and how is it related to confidence intervals?
ANOVA (Analysis of Variance):
- Define analysis of variance (ANOVA) and its components.
- Discuss the one-way ANOVA and its assumptions.
- Interpret the results of an ANOVA test.
- Explain post-hoc tests used after ANOVA.
- Discuss the limitations of ANOVA and its alternatives.
- Discuss the assumptions of ANOVA and how to check them.
- Describe the F-test and its applications in ANOVA.
- Explain the concept of interaction effects in factorial ANOVA.
- Discuss the post hoc tests used in ANOVA, such as Tukey’s HSD and Bonferroni correction.
Chi-Square Test:
- Define the chi-square test and its applications.
- Explain the chi-square test for independence.
- Calculate the expected frequencies for a chi-square test.
- Interpret the results of a chi-square test.
- Discuss the assumptions of the chi-square test.
- Define the chi-square test and its applications in contingency tables.
Bayesian Statistics:
- Define Bayesian statistics and its principles.
- Explain Bayes’ theorem and its applications.
- Discuss the concept of prior and posterior distributions.
- Explain the concept of prior and posterior distributions in Bayesian inference.
- Describe Markov Chain Monte Carlo (MCMC) methods.
- Discuss the advantages and limitations of Bayesian statistics.
- Discuss the difference between frequentist and Bayesian approaches to statistical inference.
This list (important questions of statistics) covers a wide range of topics in statistics commonly included in postgraduate entrance exams. Each topic contains multiple questions of statistics to provide a comprehensive overview of the subject matter.
Copyrighted Material © 2019 - 2024 Prinsli.com - All rights reserved
All content on this website is copyrighted. It is prohibited to copy, publish or distribute the content and images of this website through any website, book, newspaper, software, videos, YouTube Channel or any other medium without written permission. You are not authorized to alter, obscure or remove any proprietary information, copyright or logo from this Website in any way. If any of these rules are violated, it will be strongly protested and legal action will be taken.
Be the first to comment